Identify depression
using AI
Below is a free classifier to identify depression. Just input your text, and our AI will predict if it indicates depression or not - in just seconds.
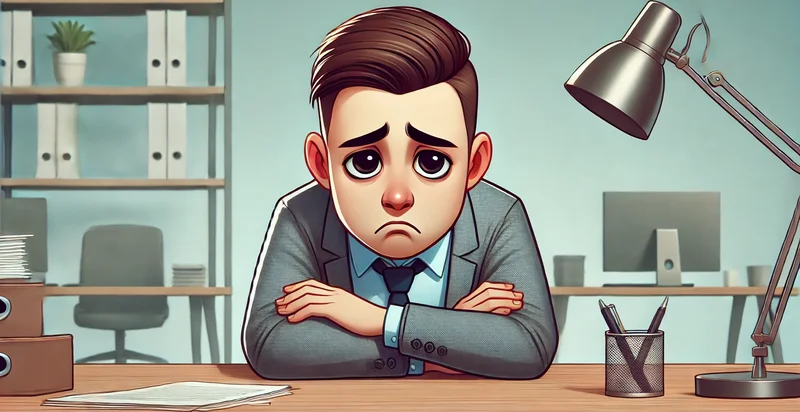
API Access
import nyckel
credentials = nyckel.Credentials("YOUR_CLIENT_ID", "YOUR_CLIENT_SECRET")
nyckel.invoke("depression-identifier", "your_text_here", credentials)
fetch('https://www.nyckel.com/v1/functions/depression-identifier/invoke', {
method: 'POST',
headers: {
'Authorization': 'Bearer ' + 'YOUR_BEARER_TOKEN',
'Content-Type': 'application/json',
},
body: JSON.stringify(
{"data": "your_text_here"}
)
})
.then(response => response.json())
.then(data => console.log(data));
curl -X POST \
-H "Content-Type: application/json" \
-H "Authorization: Bearer YOUR_BEARER_TOKEN" \
-d '{"data": "your_text_here"}' \
https://www.nyckel.com/v1/functions/depression-identifier/invoke
How this classifier works
To start, input the text that you'd like analyzed. Our AI tool will then predict if it indicates depression or not.
This pretrained text model uses the Depression in Social Media Texts dataset and has 2 labels, including Likely Depressed or Likely Not Depressed.
We'll also show a confidence score (the higher the number, the more confident the AI model is around if it indicates depression or not).
Whether you're just curious or building depression detection into your application, we hope our classifier proves helpful.
Recommended Classifiers
Need to identify depression at scale?
Get API or Zapier access to this classifier for free. It's perfect for:
- Mental health screening: Analyze patient messages for signs of depression. Prioritize individuals who may need immediate care.
- Social media monitoring: Identify users potentially at risk for depression. Provide supportive resources and helpline information.
- Employee wellness programs: Screen internal communications for mental health concerns. Offer targeted support to staff who may be struggling.
- Healthcare chatbots: Detect depressive language in user interactions. Escalate cases to human professionals when necessary.
- Research studies: Analyze large text datasets for depression indicators. Identify trends and patterns in mental health expressions.
- Crisis hotline triage: Assess incoming messages for severity of depression. Route high-risk cases to specialized counselors quickly.
- Educational institutions: Monitor student writings for signs of depression. Intervene early to support at-risk students' mental health.