11 Roboflow Alternatives & Competitors
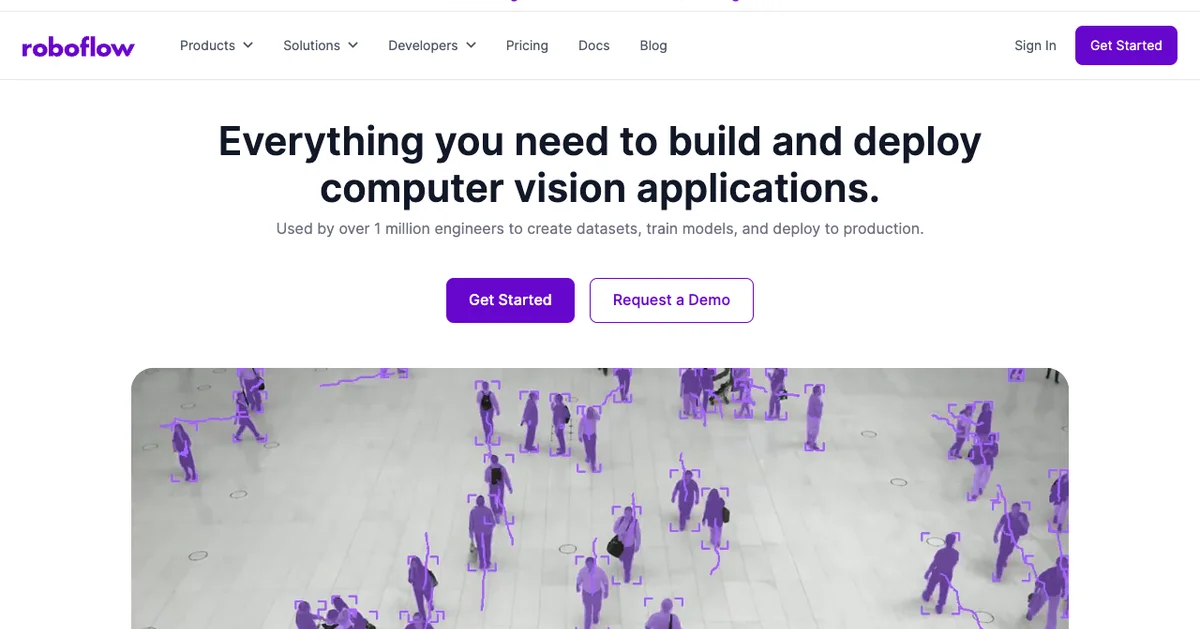
Computer vision developers rely on Roboflow to build and deploy applications without getting bogged down in technical complexities. The platform has proven its worth across 16,000+ organizations, streamlining machine learning workflows through seamless integration with common open-source libraries. For teams starting new AI projects, Roboflow provides the essential building blocks while eliminating many traditional development hurdles.
The growth of computer vision applications has created more need for developer-friendly tools that deliver results. Roboflow stands out by making image and video analysis accessible through straightforward features that support everything from basic classification to advanced object detection. While the platform works well for many use cases, developers should evaluate other available options based on their project’s specific technical needs and constraints.
While Roboflow is a great product, it’s always good to shop around and look for alternatives. This article identifies some top competitors and their pros and cons.
Table of Contents
- Nyckel
- TensorFlow
- Amazon Rekognition
- Google Cloud Vision AI
- IBM Watson Visual Recognition
- Microsoft Azure Computer Vision
- Clarifai
- Scikit-image
- Viso Suite
- OpenAI
- OpenCV
Nyckel
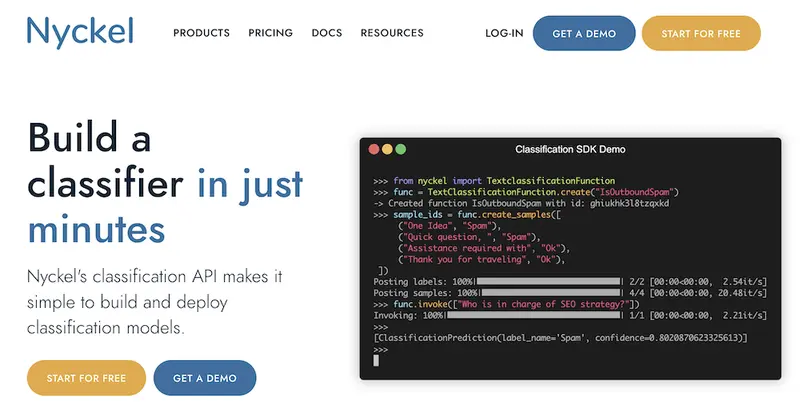
Nyckel makes it easy to build custom machine learning models for everything from classification to detection to elastic search. Coupled with their in-house ML experts who can help scope your project, they allow you to design the exact ML platform you want, without having to hire an in-house ML team.
Unlike traditional machine learning tools, Nyckel abstracts the commoditized aspects of a ML model: hosting, model and algorithm selection, deployment, and maintenance. Creating a high-accuracy model is as easy as uploading as few as 5-10 training samples, and then waiting less than a minute for the platform to train.
Pros
- Much more accurate than LLMs
- Train a model with as few as 5 samples per class
- Model takes ~30s to train
- Annotations update the model in real-time (~5s)
- Easy to see what’s being invoked, which can be used for fine-tuning
- Offer text and tabular data models as well
Cons
- Don’t have full visibility into the models used
- Can’t adjust any weights and biases yourself
TensorFlow
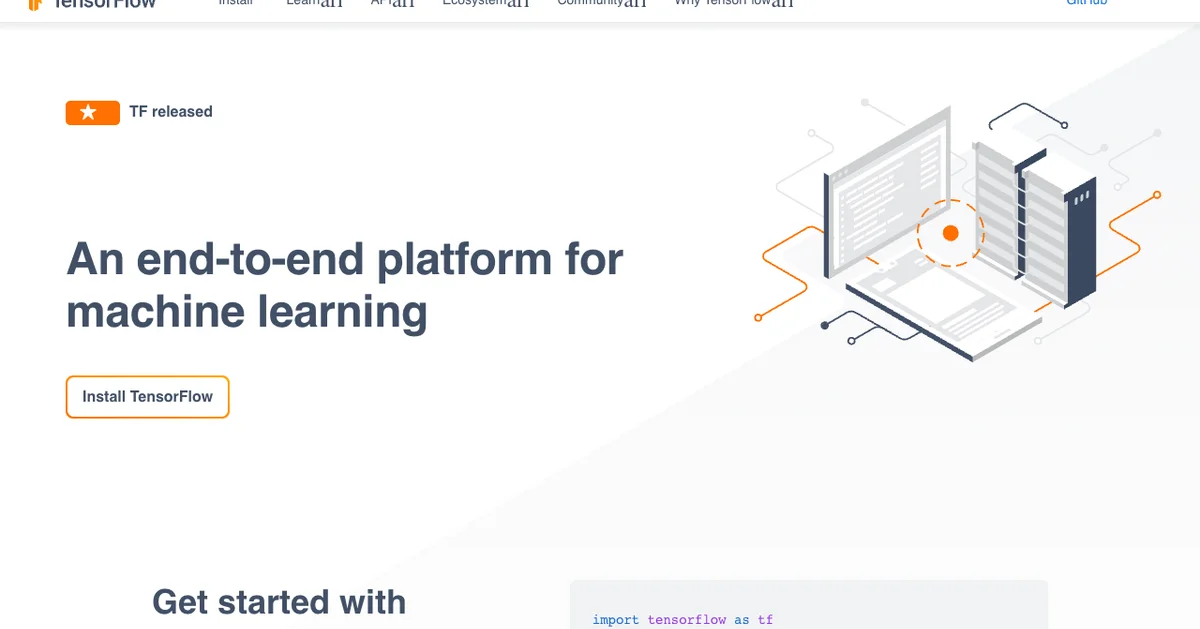
TensorFlow stands as a robust machine learning framework born from Google Brain’s research team. While some platforms like Roboflow zero in on computer vision, TensorFlow casts a wider net across machine learning applications. The framework powers everything from desktop applications to mobile devices, handling tasks from natural language processing to reinforcement learning.
The framework shines through its adaptable architecture. Teams can choose between Keras for rapid prototyping or work with lower-level APIs when precision matters. Real-world deployments span cloud services, local servers, and edge devices, making it particularly valuable for growing projects. The active developer community and detailed documentation create a support system that serves both newcomers and seasoned practitioners.
Data handling capabilities set TensorFlow apart from alternatives. Built-in dataset tools streamline access to training data, while TensorBoard brings clarity to complex model behaviors through visual analytics. These features prove especially valuable when working with large-scale projects where performance optimization becomes critical.
TensorFlow demonstrates particular strength in projects requiring broad machine learning capabilities beyond standard computer vision tasks. The platform continues to evolve, backed by both Google’s resources and community contributions.
Pros
- Comprehensive machine learning toolkit
- Multiple API layers for different skill levels
- Rich documentation and community support
- Flexible deployment options
- Advanced visualization capabilities
Cons
- Steeper learning curve for new users
- More initial configuration needed
- Features may exceed simple project needs
Amazon Rekognition
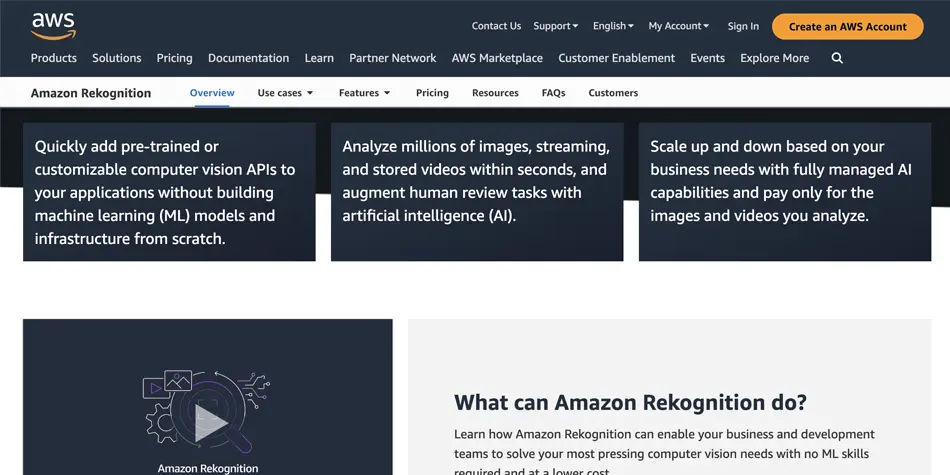
Amazon Rekognition brings machine learning-powered image and video analysis to the AWS platform. The service enables developers to incorporate visual analysis into their applications, handling everything from facial recognition to object detection, without requiring extensive ML expertise.
Rekognition excels at processing images and video footage in real-time, making it particularly valuable for security monitoring, customer analytics, and content filtering. Organizations can leverage its automated tagging system to streamline media management. The facial matching capabilities have proven especially useful for access control and identity verification across various industries.
The service runs on Amazon’s extensive cloud infrastructure, allowing seamless scaling as usage demands increase. This makes it particularly suitable for enterprises expecting substantial growth in their visual analysis needs. Deep integration with other AWS tools like S3 storage reduces development complexity and time-to-market.
Compared to Roboflow’s data preparation focus, Rekognition delivers comprehensive visual analysis capabilities right out of the box. The platform stands apart through its enterprise-grade infrastructure and ability to handle large-scale deployment needs.
Pros
- Seamless AWS ecosystem integration
- Advanced image and video processing
- Enterprise-grade scalability
- Real-time analysis capabilities
Cons
- Requires AWS platform knowledge
- Limited custom model development options compared to Roboflow
Google Cloud Vision AI
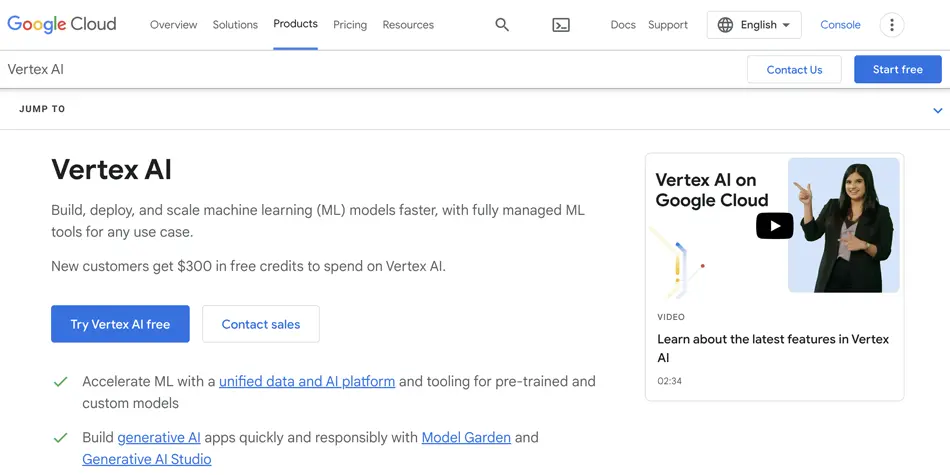
Google Cloud Vision AI delivers advanced image recognition capabilities as part of Google’s cloud platform. The service processes images to identify objects, extract text, and categorize visual content - making it valuable for organizations that need robust computer vision capabilities in their tech stack.
The platform shines through its native compatibility with Google Cloud’s broader ecosystem. Organizations already running Google services can improve their existing workflows by adding image analysis features. Built on Google’s machine learning infrastructure, the system continuously evolves as new models and capabilities roll out.
Google developed Cloud Vision AI to change how businesses work with visual data at scale. Manufacturing companies use it to spot defects on production lines, while retailers implement it to improve product discovery. The technology opens up possibilities across sectors, from healthcare imaging to content moderation.
The straightforward interface makes computer vision accessible to teams without specialized expertise. Comprehensive guides and resources help organizations implement the technology effectively, though some initial learning investment is needed.
Pros
- Native integration across Google Cloud platform
- Robust machine learning foundation
- Regular feature and model updates
- Clear documentation and intuitive controls
Cons
- Initial complexity for new users
- Deep ties to Google services limit flexibility
- Usage costs can accumulate quickly
IBM Watson Visual Recognition
IBM Watson Visual Recognition combines decades of AI expertise with modern computer vision capabilities. The platform stands as a compelling alternative to Roboflow, particularly due to its seamless IBM Cloud integration. This infrastructure supports everything from small-scale implementations to complex enterprise deployments, giving teams the flexibility to scale as needed.
The system demonstrates particular strength in image analysis tasks, from basic object detection to nuanced content filtering. Organizations can build custom models using their specific image collections, making the platform adaptable across different industries and use cases. This customization has proven especially valuable for businesses needing specialized recognition capabilities.
Behind the technology lies IBM’s established track record in enterprise computing and commitment to responsible AI development. Their emphasis on security and transparency resonates strongly in today’s privacy-conscious market. The recent AWS partnership has further strengthened their offering, particularly for organizations prioritizing data protection and system reliability.
The platform comes backed by extensive documentation and learning materials, enabling teams to build competency while implementing solutions.
Pros
- Deep integration with IBM Cloud infrastructure
- Flexible custom model development options
- Robust learning resources and technical support
- Strong focus on ethical AI development
- Proven enterprise-grade reliability
Cons
- Complex initial setup process
- Premium pricing structure
- Enterprise focus may not suit smaller teams
Microsoft Azure Computer Vision
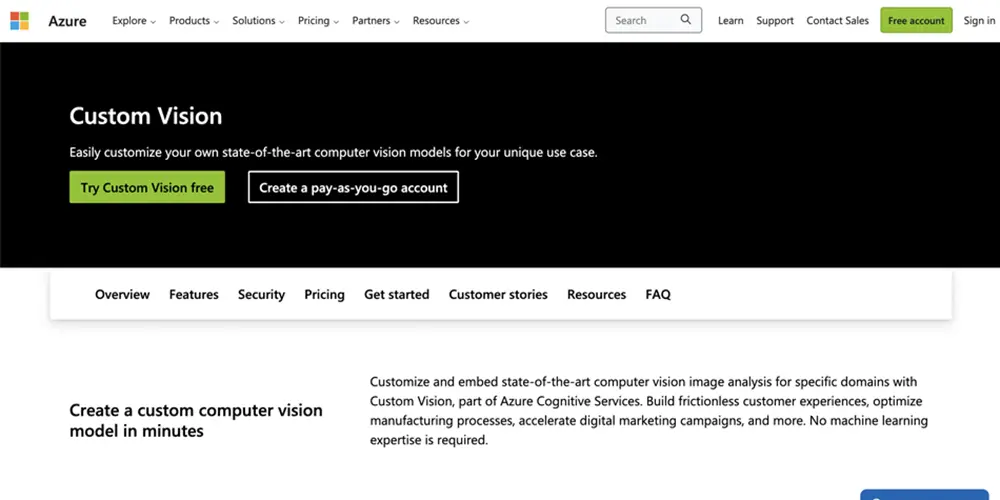
Microsoft Azure Computer Vision delivers sophisticated image and video analysis through Azure’s cloud platform. Built for development teams needing visual recognition tools, it processes images to identify objects and extract text using machine learning technology.
The platform stands out with its automatic image description capabilities, enabling companies to improve user experiences through detailed image content analysis. Video recognition features add another dimension, tracking activities and movements - particularly valuable for monitoring systems and retail analytics.
Built on Microsoft’s established cloud infrastructure, Azure Computer Vision scales effectively as usage grows. Organizations already using Azure services find the integration straightforward, with APIs and SDKs that connect smoothly to existing workflows.
Regular platform updates keep the technology current with emerging computer vision advances. Enterprise-grade security protocols and compliance standards make it suitable for organizations dealing with sensitive visual data.
Azure Computer Vision presents a compelling option compared to Roboflow, particularly for teams wanting Azure ecosystem integration alongside robust image and video processing capabilities.
Pros
- Advanced image and video processing tools
- Smart image description functionality
- Seamless Azure service compatibility
- Regular platform enhancements and security
Cons
- Steep learning curve for new users
- Premium pricing affects smaller operations
- Microsoft account requirement
Clarifai
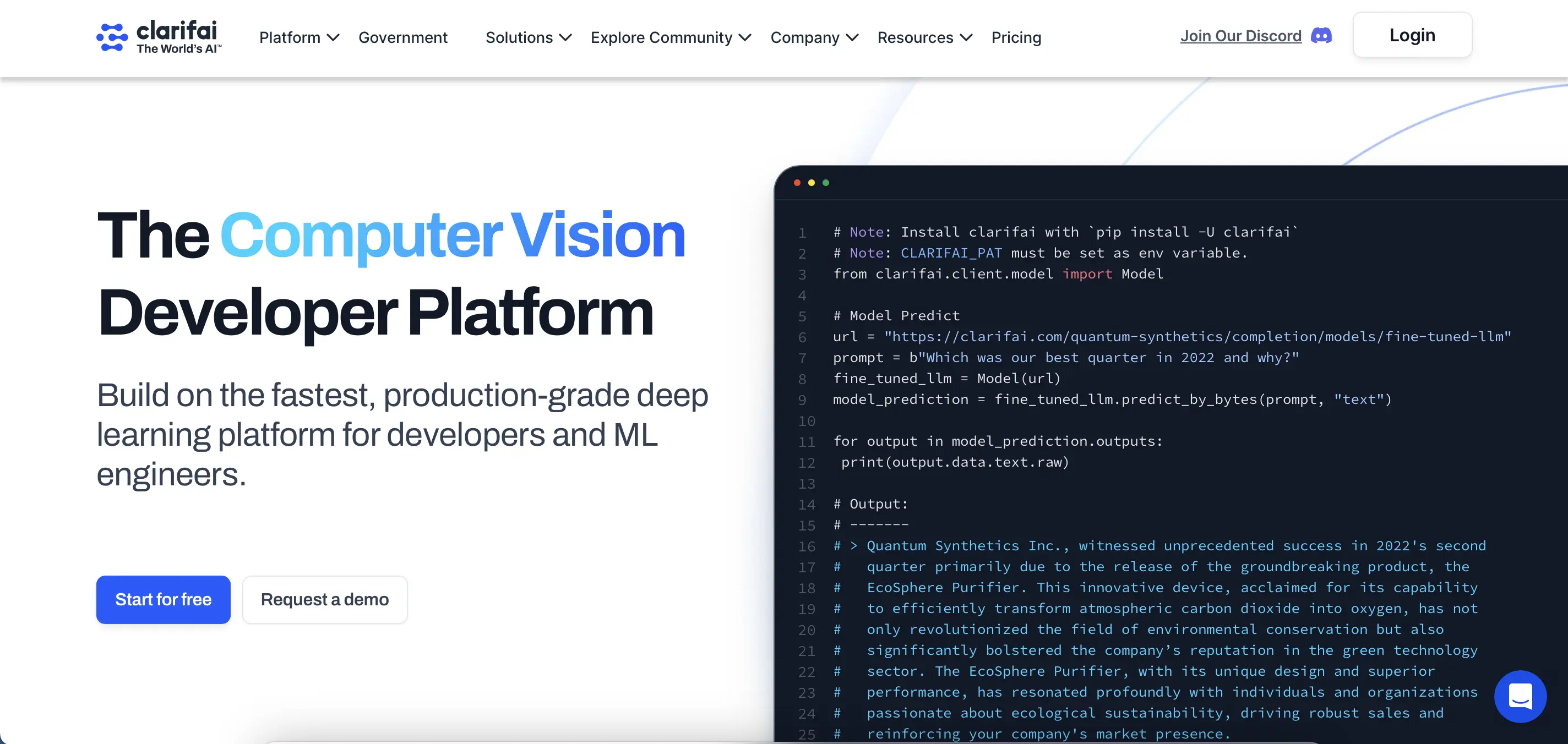
Clarifai stands out in the AI landscape with its specialized computer vision capabilities. Their AI lifecycle platform bridges the gap between initial concepts and full-scale deployment, streamlining the development process. By providing a complete ecosystem for building and managing AI applications, Clarifai enables teams to standardize their approach and roll out production-ready solutions much faster than traditional methods.
The platform excels at automatically processing and labeling various data types - from images to video content. This functionality proves invaluable for organizations drowning in unstructured data who need quick, actionable insights. Built-in content monitoring and moderation systems add another layer of practical value, helping businesses maintain their standards while navigating compliance requirements.
Recent platform updates have brought Large Language Models and Generative AI into the mix, expanding Clarifai’s toolkit. Teams can now leverage both established and custom-built models to tackle content creation and analysis tasks. The addition of predictive maintenance capabilities gives organizations a heads-up on potential system issues before they impact operations.
Clarifai delivers a robust solution for organizations ready to scale their AI initiatives while keeping security and workflow management in check.
Pros
- Quick prototype-to-production deployment
- Efficient auto-labeling across multiple data formats
- Flexibility between standard and custom AI models
- Built-in predictive maintenance tools
- Strong security and compliance features
Cons
- Notable learning curve for new users
- May be too robust for smaller teams
Scikit-image
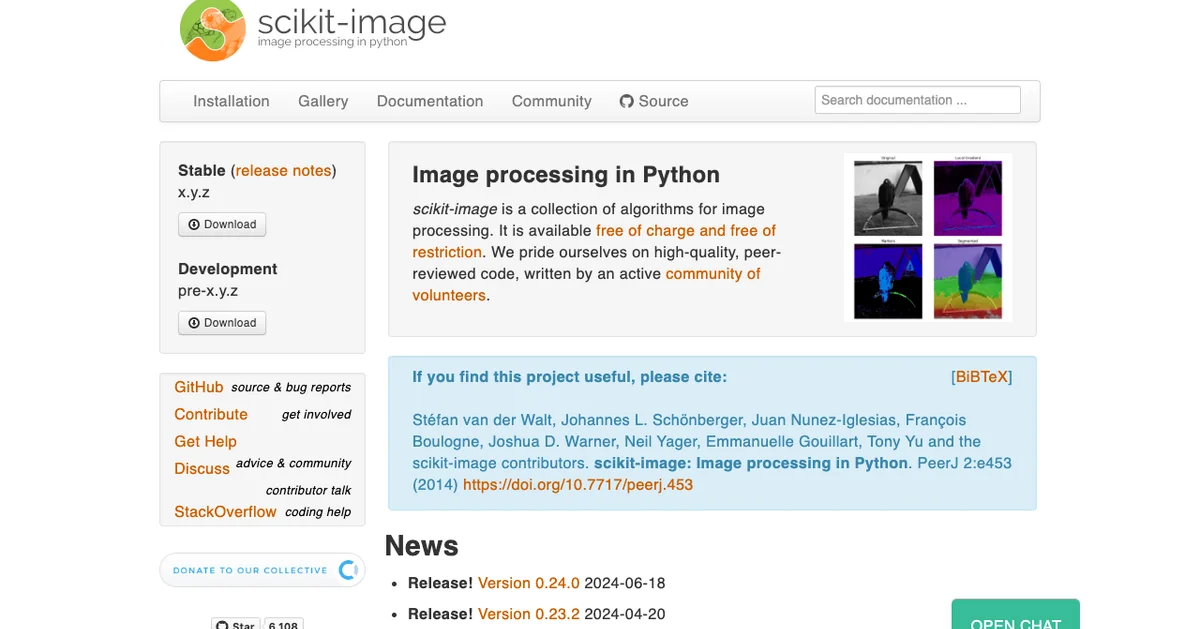
Scikit-image stands as a robust open-source library for image processing in Python. The platform serves developers and researchers who need comprehensive computer vision capabilities without commercial software costs. Built by dedicated volunteers and maintained through peer review, the library delivers professional-grade algorithms for everything from basic image manipulation to advanced analysis tasks.
The straightforward nature of Scikit-image makes complex operations surprisingly manageable. Basic tasks like image filtering, edge detection, and pixel manipulation require minimal code. Thanks to its NumPy foundation, the library meshes seamlessly with Python’s scientific computing ecosystem. This architecture gives developers room to experiment and craft specialized solutions for unique challenges.
Community involvement drives continuous improvements to Scikit-image through grant funding and developer contributions. Regular updates and enhancements reflect real-world needs from users across different sectors. The documentation includes practical examples and galleries that demonstrate key concepts, making it easier for newcomers to grasp fundamental principles and start building.
Compared to more streamlined tools like Roboflow, Scikit-image provides deeper control over image processing workflows. Developers who need precise customization and hands-on coding often gravitate toward Scikit-image for its flexibility and extensibility.
Pros
- Free and open-source
- Engaged community backing
- Sophisticated image processing capabilities
- Seamless Python ecosystem integration
- Regular feature updates
Cons
- Higher initial learning investment
- Fewer built-in convenience tools than Roboflow
- Requires programming knowledge
Viso Suite
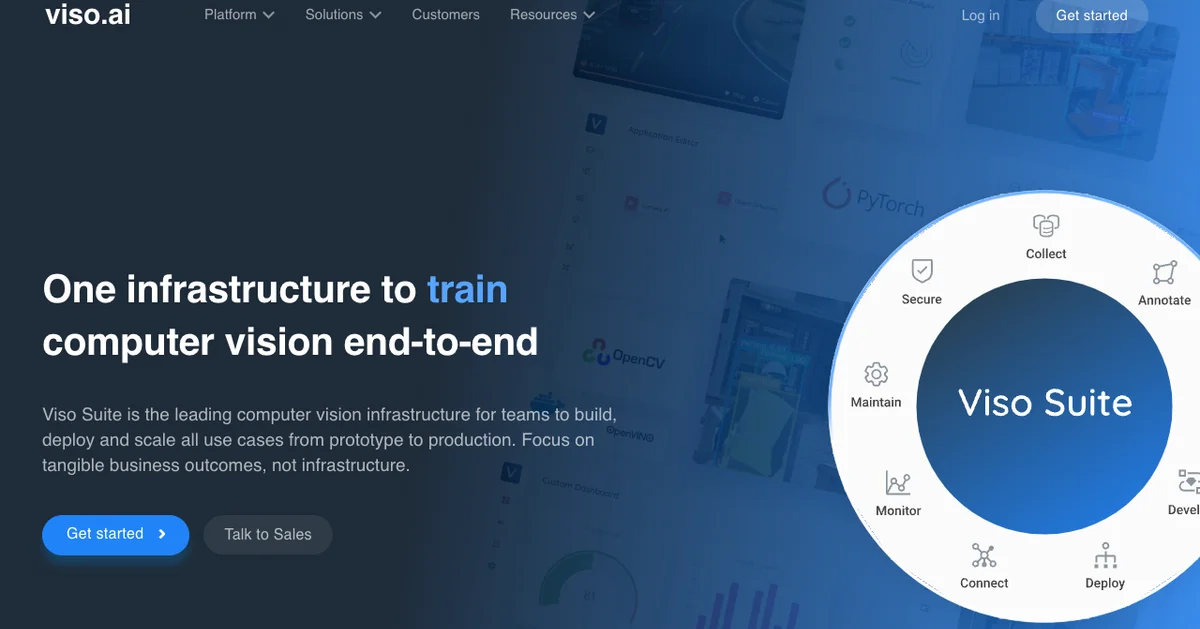
Viso Suite delivers a powerful end-to-end computer vision platform that streamlines AI vision application development and deployment. The platform tackles common infrastructure headaches while enabling teams to focus on building solutions that drive real business results.
The platform’s modular design sets it apart, giving developers the flexibility to construct efficient vision pipelines without unnecessary complexity. Teams can seamlessly integrate cameras, models, and processing components based on their specific requirements. The platform handles both pre-trained and custom models across different frameworks, centralizing everything in a single, organized workspace.
Security and data governance remain central to Viso Suite’s architecture. The automated data collection system keeps sensitive information within designated workspaces, while robust device management tools enable smooth deployment across multiple endpoints without manual configuration overhead.
Viso Suite helps technical teams to maintain full ownership of their AI initiatives. The platform bridges the gap between initial prototypes and production deployments. Built-in monitoring and analytics capabilities help teams track performance and optimize their applications based on concrete metrics.
When compared to alternatives like Roboflow, Viso Suite provides broader functionality spanning data management, model development, analytics, and device orchestration - essentially serving as a complete toolkit for enterprise computer vision.
Pros
- End-to-end platform covering the full AI application lifecycle
- Flexible modular pipeline architecture
- Improved data security and governance
- Comprehensive device orchestration
- Performance monitoring and analytics
Cons
- Notable learning curve for beginners
- Functionality may exceed small project needs
- Cost considerations for certain implementations
OpenAI
OpenAI’s computer vision capabilities, integrated into their GPT-4 model, represent a significant advancement in AI-powered image understanding. The system can analyze and interpret images with remarkable accuracy, combining visual perception with natural language understanding to provide detailed descriptions, answer questions, and perform complex visual reasoning tasks.
GPT-4 excels at interpreting a wide range of visual content, from diagrams and charts to natural scenes and technical documentation. Its multimodal capabilities allow it to understand context and provide detailed explanations about what it sees, making it particularly valuable for tasks like content description, visual analysis, and image-based problem-solving. Organizations can leverage its advanced capabilities for tasks ranging from accessibility improvements to complex document analysis.
The service operates through OpenAI’s cloud infrastructure, providing reliable and consistent performance through their API. This makes it especially suitable for businesses looking to integrate sophisticated visual understanding into their applications without managing complex ML infrastructure. The system’s ability to handle diverse image types and provide natural language responses makes it particularly accessible to developers without extensive computer vision expertise.
Pros
- Reliable infrastructure
- Transparent pricing; pay-as-you-go only
- Good zero-shot accuracy
Cons
- Difficult to fine-tune and improve accuracy
- Can be very expensive at scale (millions of API calls a month)
OpenCV
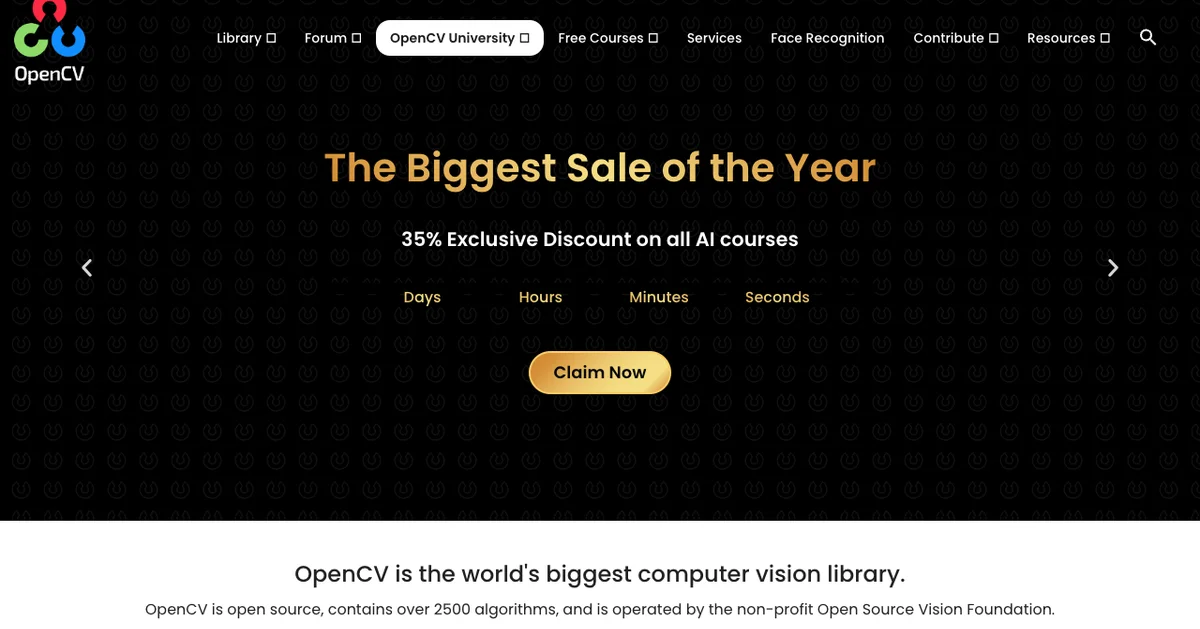
OpenCV, or Open Source Computer Vision Library, stands out as one of the largest and most comprehensive computer vision resources available. It’s an open-source project managed by the non-profit Open Source Vision Foundation, which nurtures a vibrant community of developers and users. Over 2,500 algorithms are offered through OpenCV, catering to a range of tasks such as image processing, facial recognition, and machine learning. Its extensive capabilities make it appealing for both beginners and experienced professionals looking to explore computer vision.
One of OpenCV’s unique features is its optimization for real-time applications, making it suitable for systems that require rapid response times. Its cross-platform functionality allows it to function seamlessly on various operating systems like Linux, MacOS, and Windows, as well as mobile platforms such as iOS and Android. This flexibility gives developers the freedom to implement solutions across diverse environments.
OpenCV isn’t just a powerful library; it’s also a hub for learning. With a strong emphasis on education, OpenCV offers courses, tutorials, and a supportive community forum. This educational aspect sets it apart from Roboflow, which primarily focuses on providing tools for model development and deployment. OpenCV’s commitment to fostering understanding in computer vision can enhance the skills of users and make it more accessible.
OpenCV’s emphasis on open-source software is another standout feature. It allows developers to modify and adapt the library to their own needs, which can be particularly valuable in specialized projects.
Pros
- Open-source and free for commercial use
- Extensive library with numerous algorithms
- Real-time application optimization
- Cross-platform support
- Strong educational resources and community
Cons
- May require more technical expertise to fully leverage
- Lacks some user-friendly tools found in other platforms like Roboflow